Mathematical modelling of multi-physics fluids
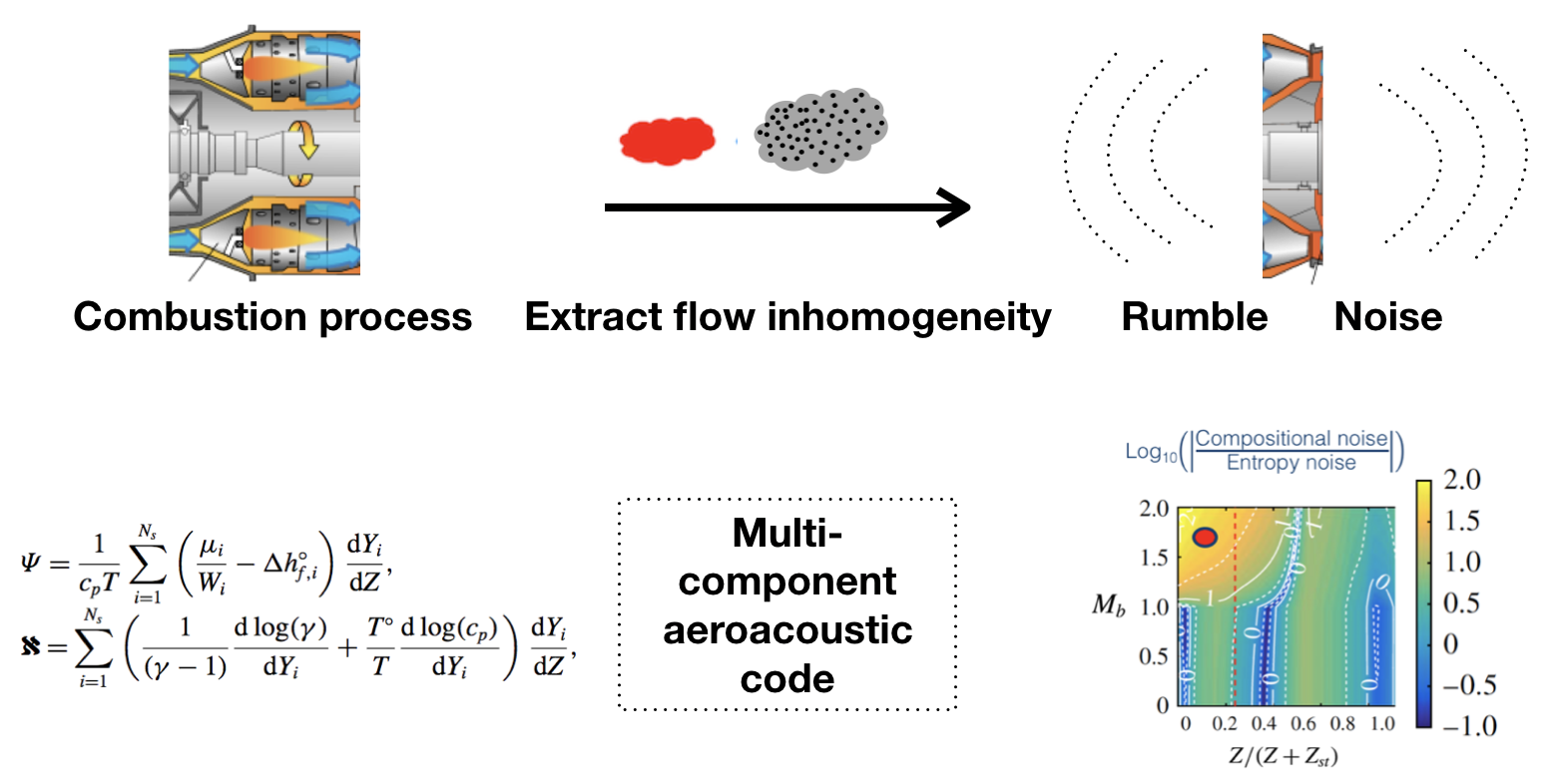
The combustion process generates flow inhomogeneities because it is turbulent and mixing is not fully completed. The flow inhomogeneities are extracted from the exit of the combustor and are taken as inputs of a reacting multi-component aeroacoustic solver, which provides the sound generated by the nozzle. Bottom-left equations from Magri (2017), J. Fluid Mech., vol. 828, R2 (Rapids). Bottom-right figure from Magri, O'Brien, Ihme (2016), J. Fluid. Mech, vol. 799, R4 (Rapids).
After fan and jet noise, combustion noise is a dominant cause of noise pollution generated by the whole aeroengine. Although methods to mitigate fan and jet noise have been in place for some time - such as ultra-high bypass ratio turbofan engines, acoustic liners and fan blade geometric design - combustion noise is bound to increase with the implementation of low-emission combustors. Combustion noise can be caused by direct and indirect mechanisms.
Direct combustion noise is caused by the heat released by the unsteady flame, which is a powerful monopole source of sound. The acoustics emitted by it propagate in the combustion chamber through the turbine and are distorted by mean-flow gradients. The sound that is transmitted through the downstream-engine component causes noise pollution, whereas the sound that is reflected off at the nozzle can create a thermoacoustic feedback. Indirect noise is caused by the interaction between flow inhomogeneities exiting the combustion chamber and being accelerated through the nozzle downstream of the combustor. Temperature, vorticity and compositional inhomogeneities are sources of indirect noise. Studies showed that indirect mechanisms are crucial for the analysis of combustion noise and thermoacoustic instability. |